Use cases
Every operator manages processes that feel totally unique to their organization — rich with messy data coming from every source imaginable. The good news is that if it can be done manually, it can be automated in Parabola.
In our use case library, learn about processes that can (rather, should) be automated and see how other operators are solving similar problems through customizable "Templates."
Featured use cases
AI-enabled use cases
Explore other common use cases
Inventory replenishment monitoring
Inventory replenishment monitoring, Operations, Customer support, Supply chain, Shopify, Amazon Seller Central, Email, low inventory, stock, out of stock, OOS, alerting, Slack, email, Order & Inventory management, Reporting & Forecasting, Alerts & Monitoring
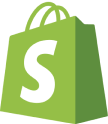

TEMPLATE AVAILABLE
Wholesale OTIF fulfillment scorecarding
Wholesale OTIF fulfillment reporting, Supply chain, Operations, Netsuite, 663559c9207f91d5a1baa251, Amazon Seller Central, Walmart, Ulta, on-time, in-full, on time, in full, fill rate, scorecard, Reporting & Forecasting, Order & Inventory management, PO & Invoice Automation

TEMPLATE AVAILABLE
There are no results, please try another search.